Biostatistics Shared Resource
Please remember to cite the Shared Resources!
Research reported in this publication was supported by The Ohio State University Comprehensive Cancer Center and the National Institutes of Health under grant number P30 CA016058.
We thank the XX Shared Resource at The Ohio State University Comprehensive Cancer Center, Columbus, OH for (XX)
About Us: (back to top)
The Biostatistics Shared Resource (BSR) enables OSUCCC – James researchers to collaborate on all aspects of experimental design; grant proposal development; data management and statistical analysis of clinical, epidemiological, public health and laboratory research data; and manuscript preparation
250 Lincoln Tower
1800 Cannon Dr.
Columbus, OH 43210
Phone: 614-292-4778
Fax: 614-688-6600
biostatistics@osumc.edu
Availability: Monday-Friday, 8 a.m.-5 p.m.
For more information, please contact us at biostatistics@osumc.edu. If you need assistance with a specific project, please use our support request form.
Meet the Team: (back to top)
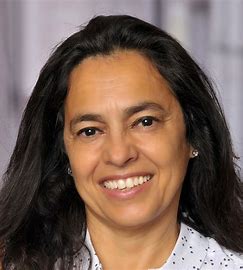
Disease Group |
Biostatistical Navigator(s) |
Breast | Julie Stephens and Marilly Palettas |
Central Nervous System | Jeff Pan |
Head & Neck | Sonia Zhao |
Thoracic | Lai Wei and Sonia Zhao |
Sarcoma | Xiaoli Zhang |
Genitourinary | Jeff Pan |
Thyroid/Neurology | Lai Wei |
Gastrointestinal | Mohamed Elsaid |
Melanoma | Xiaoli Zhang |
Gynecologic | Rachel Smith |
Leukemia and Bone Marrow Transplant | Lai Wei and Eric McLaughlin |
Myeloma | Xiaoli Zhang |
Lymphoma | Lai Wei |
Translational Therapeutics | Julie Stephens |
Cancer Control | Mohamed Elsaid |
Cancer Biology | Lianbo Yu |
Molecular Carcinogenesis and Chemoprevention | Molly Mo |
Leukemia Research | Lai Wei |
Available Services: (back to top)
Click here for full list of services and fees
Design and Analysis
Center biostatisticians focus on study design and planning as their most critical contribution to collaboration. This requires frequent interactions that result in improvements in experimental design, convincing conclusions and revealing data analyses.
Our expertise in design and analysis includes:
- Multiple hypothesis testing strategies
- Laboratory experimental design
- Bioassay experiments
- Robust mixed modeling for experimental data with dependency structure
- Cancer control design and analysis
- Propensity score matching for observational study designs
- Complex modeling for longitudinal and other observational studies
- Re-estimation of sample size
- Evaluation of diagnostic techniques
Clinical Trials
Center biostatisticians work with OSUCCC – James principal investigators on clinical trials conducted in cancer prevention, detection, diagnosis and treatment.
Our expertise in clinical trials includes:
- Bayesian designs
- Interim analyses and monitoring
- Development of study design and decision rules
- Choice of outcome measures
- Sample size and power calculations
- Development of case report/data collection forms
- Analysis of correlative data including pathway analysis
- Chemoprevention trials
- Re-estimation of sample size
- Repeated measures designs including crossover and semi-crossover
High Dimensional Data Analysis Support
There are seven biostatisticians in the Center who have expertise in the design and analysis of studies with high dimensional data.
Our expertise in high dimensional data analysis support includes:
Statistical Methods:
- Technical Noise Filtering
- Sample normalization
- Robust variance estimation via hierarchal modeling
- Moderated T-test for differential expression
- Dimension reduction
- Data visualization
- Integration of multiple data types
- Clustering and classification
- Prognostic and diagnostic multivariate modeling
- Gene ontology and pathway analysis
Design Issues:
- Sample size to control power distribution
- Methods of controlling false discoveries
- Feature selection
- Biomarker discovery and validation
Data Type Experience:
- Bulk RNA-Seq
- Single-Cell RNA-Seq
- ChIP-Seq
- NanoString
- SNP/CNV
- GWAS
- Proteomics
- Microbiome
- Methylation array
- Affymetrix array
- Customized array
New Methods
Early Phase Clinical Trial Adaptive Design: Barriers with implementation of adaptive designs and development of statistical methods for adaptive phase I/II designs
Adaptive Sample Size:
Developed a re-estimation method that retains blinding of group assignment and allows for an increase in enrollment when the event rate (recurrence for one trial, death for the other) is lower than initially anticipated (Clinical Trials, 2010). This method provides blinded re-estimation and bootstrapped sample size distributions, which provides confidence in settling on a sample size near the end of planned accrual.
Adaptive Design for Laboratory Experiments:
Adapted the group sequential design to develop a novel approach for two-stage adaptive laboratory experiments for small sample sizes (Statistics in Biosciences, 2015). This method allows small sample size approach to designing a subsequent experiment that controls overall type I error and achieves sufficient conditional power.
Methods for Donor Cell Experiments:
Donor cell experiments involve repeated measures of the cells from the same donor receiving different treatments. The BSR has developed a method of robustly testing hypotheses in these experiments by using concepts from conditional mixed models to ensure unbiased estimation of variance and precise type I error control. This method avoids estimating a saturated co-variance structure, which is often computationally difficult due to small sample size, by defining appropriate variance components for specific contrasts of the fixed effects.
Mechanism Hypothesis Testing:
Many cell experiments involve up-and-down regulation to validate direct control of the target outcome. This requires significant differences for both up-and-down conditions vs. control. The BSR has identified a method of determining sample size that ensures, with chosen power, significance for both comparisons simultaneously. The same method is used when testing a combination of conditions against each condition alone. Similarly, the BSR is researching methods to draw broader conclusions from a series of experiments that add confidence to a hypothesis about pathway mechanisms compared to more limited conclusions for each experiment in a series.
Power Analysis for RNA-Seq Differential Expression Studies:
We developed a simulation-based procedure for power estimation using the negative binomial distribution for RNA-seq studies. This approach assumes a generalized linear model (at the gene level) that considers the dependence between gene expression level and its variance (dispersion) and also allows equal or unequal dispersion across conditions (BMC Bioinformatics, 2017).
Please remember to cite the Shared Resources!
Research reported in this publication was supported by The Ohio State University Comprehensive Cancer Center and the National Institutes of Health under grant number P30 CA016058.
We thank the XX Shared Resource at The Ohio State University Comprehensive Cancer Center, Columbus, OH for (XX)